Guide to Data-Driven Decision Making- Process, Tips & Benefits
Big data is perhaps the biggest buzzword of the past decade, and for good reason. Data is central to the way businesses operate today, so much so that according to the International Data Corporation, spending on data and analytics is predicted to reach $274.3 billion by 2022.
Much of this has to do with how easy it has become to collect digital information and leverage it into actionable business intelligence. This, in turn, has helped to promote a change in thinking among business leaders, many of whom have gone from simply relying on intuition to employing data-driven decision-making processes.
Data-Driven Decision Making Explained
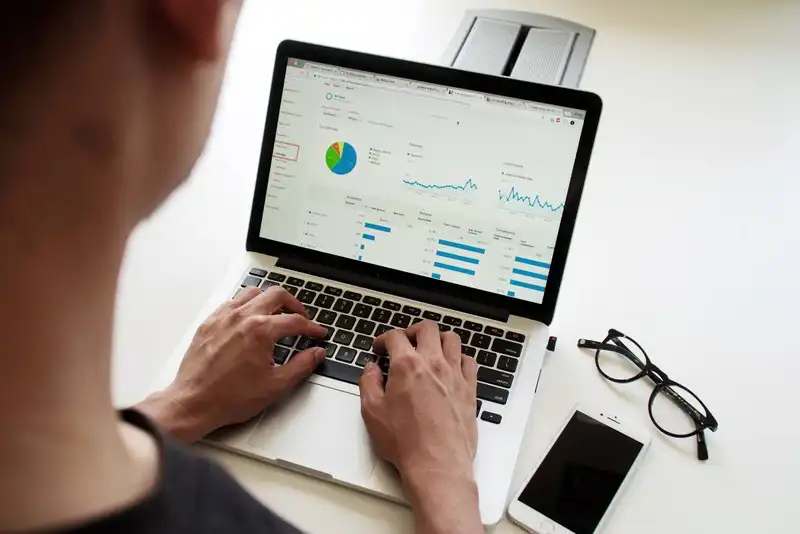
While intuition can be useful in certain situations, it would be foolish to base all business decisions on instinct alone. After all, gut feeling can't be recorded, verified, and quantified. For that, businesses need data analytics.
Data-driven decision-making (DDDM) is essentially the process of making business decisions based on actual quantifiable data instead of guesswork alone. It comes on the heels of the emergence of data analytics technologies, which have made business intelligence more accessible to non-data analysts.
This trend, known as data democratization, has unlocked more opportunities for businesses to collect information from their activities, ranging from inventory and procurement to customer service and sales among many other departments. More importantly, companies can use this data to make business decisions without requiring extensive IT support and infrastructure.
DDDM typically involves the following activities-
- Collecting data based on metrics and key performance indicators (KPIs)
- Identifying and analyzing patterns from the data
- Using the insights to develop strategies and activities to improve business operations and achieve business goals
DDDM doesn't just look good on paper, but it also generates results. A PwC survey of more than 1,000 senior executives found that highly data-driven organizations are three times more likely to be effective at decision-making than those who chose not to utilize their data.
5 Steps for Making Data-Driven Decisions
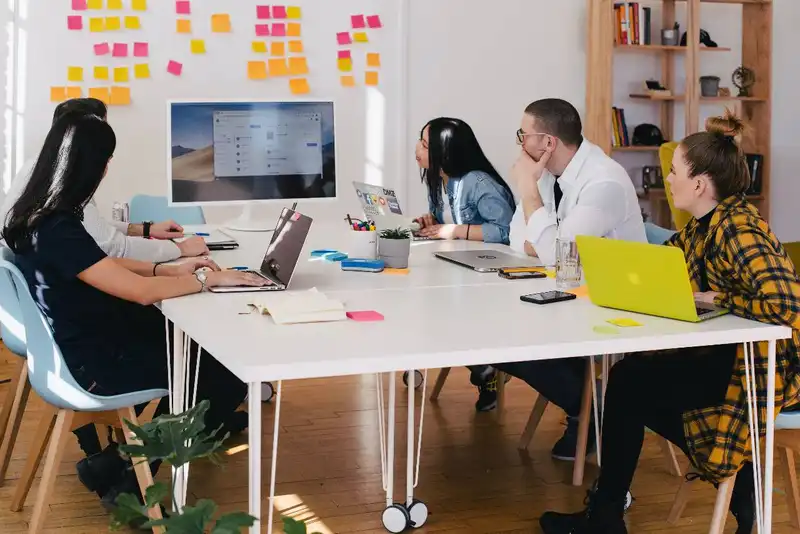
While there is no single accepted methodology for making data-based decisions, most organizations tend to follow these steps when leveraging business intelligence.
1. Set Goals for Data Analysis
An effective data analyst is someone who understands the organization and its overarching business goals. Start by asking questions such as-
- What are the most pressing problems unique to the industry? To the organization?
- What challenges does the company regularly face?
- What are the company's primary business goals?
- How can data collection and analysis help the company achieve these goals?
Knowing the answers to these questions establishes a foundational knowledge that helps the company understand the relationship between data and its business objectives. It also allows the organization to streamline its data collection methods and avoid wasting time and resources.
2. List Data Sources
Next, identify the sources from where the company will be collecting data. This can involve anything from existing databases (e.g., inventory, suppliers, newsletter subscribers) to feedback forms from consumers.
Businesses must coordinate their data sources by finding commonalities between variables of different datasets. Of course, this is easier said than done as it is much more expedient to use data for its current purposes. However, this same dataset could be used for other projects in the future.
For example, a business with an unusually high rate of employees resigning within a year of onboarding can infer that something may be wrong within its managerial and supervisor teams. But this same dataset can also be used to improve the company's recruitment practices, perhaps by improving job descriptions to better manage expectations.
3. Clean Up the Data
The advent of data analytics has made it easier than ever to collect massive volumes of data. But this is just the first step. The next hurdle is cleaning up and organizing that data for analysis.
According to IBM, organizing data can easily take up 80% of a data analyst's time, with only 20% spent on actual analysis - a problem referred to as the 80/20 rule. This underscores the importance of having clean and organized data before attempting to interpret its meaning to the business.
A reliable data analytics platform will make short work of this. But data cleaning can also be done manually on a spreadsheet program. Once datasets have been organized and cataloged in tables, simply create a data dictionary - a repository of information such as the meaning of data, the relationship between data sets, the origin of figures, and data formats.
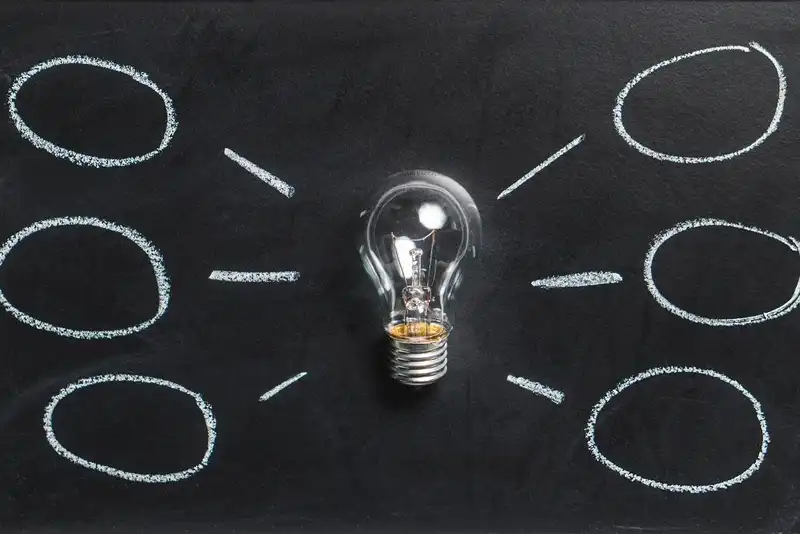
4. Conduct Statistical Analysis
After the data has been cleaned and organized, the company's data analysts can begin building statistical models to test the accuracy of data and confirm hypotheses/questions identified in the earlier stages of the data collection process. Examples of statistical models include decision trees, regressions, and random forest modeling.
Whatever model is used, the data analysts will have to decide how best to present the information to the organization's key stakeholders. This can be done in one or more of the following ways-
- Descriptive presentation that provides quantifiable facts
- Inferential presentation that provides a contextual interpretation of the facts within a particular project
- Predictive presentation, where further action is recommended based on the interpretation of findings
5. Make Conclusions
Finally, the DDDM process ends with a conclusion based on the analysis of the data. To put it simply, this involves asking the question, What did we learn from the collection and analysis of data?
Contrary to popular belief, the conclusion need not be the discovery of something new. It can also confirm something the business already knows or suspected. For example, a retail business notices that its stock tends to run out during the holidays - November and December. But before making any changes to inventory, it decides to look at historical inventory data to determine whether this hypothesis is true or not.
It's also important to remember that findings and conclusions are only useful if they're presented effectively. This means that data analysts must also be skilled at creating narratives through techniques such as data visualization.
General Guidelines for Data-Driven Decision Making
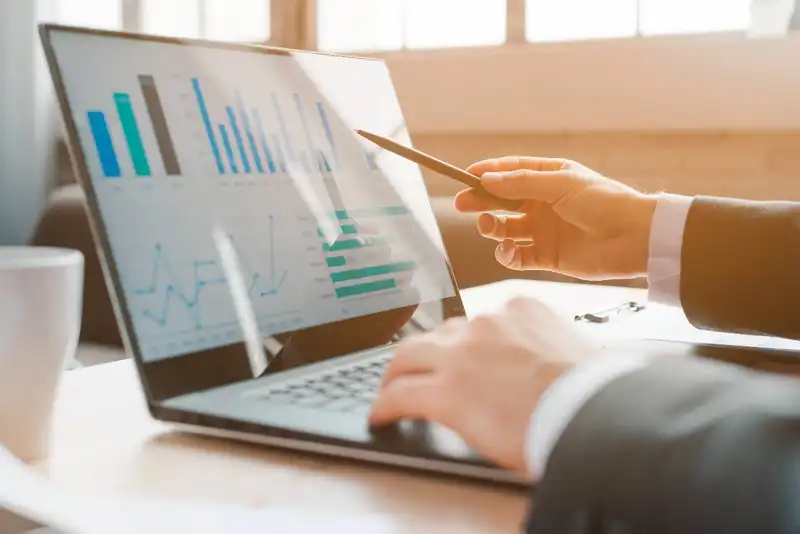
Despite the high level of interest in technology and services for data analytics, there is a disconnect between how much businesses are spending on their data analysis capabilities and how effectively they're using data in their organization. According to a survey of 64 C-level executives, only 72% said they were able to nurture a data-driven culture in their respective organizations.
This disconnect often stems from a fundamental misunderstanding of what it means to be a data-driven organization. It's not just about technology and quality data; it's also about having the right culture and internal processes to make data-driven decisions.
The following guidelines should help businesses become more effective at DDDM.
1. Check Personal Biases
Everyone has their biases, which can make it difficult to be completely objective when making data-driven decisions. As the adage goes, People see what they want to see. Fortunately, there are ways to prevent bias from contaminating data analysis and DDDM.
- Acknowledge the challenge of bias. Simply being aware that bias exists goes a long way towards reducing its impact.
- Work in teams. This ensures that data analysts can keep each other in check.
- Look for conflicting data and ask the right questions to test assumptions and initial findings.
2. Start Collecting Data as Soon as Possible
Data collection needs to happen the day the company decides to be more data-driven. There must be a conscious effort to not just collect and log information, but also to create a system for cleaning and organizing the volumes of data the business will collect.
3. Ask the Right Questions
Asking the right data analysis questions helps the team focus on relevant data, preventing them from going down rabbit holes and chasing leads that go nowhere.
This goes back to the objectives of the data analysis team. What exactly does the team want to learn from the data? What KPIs will be used to measure variables? Where will the data come from? These are just a few of the many questions data analysts should ask.
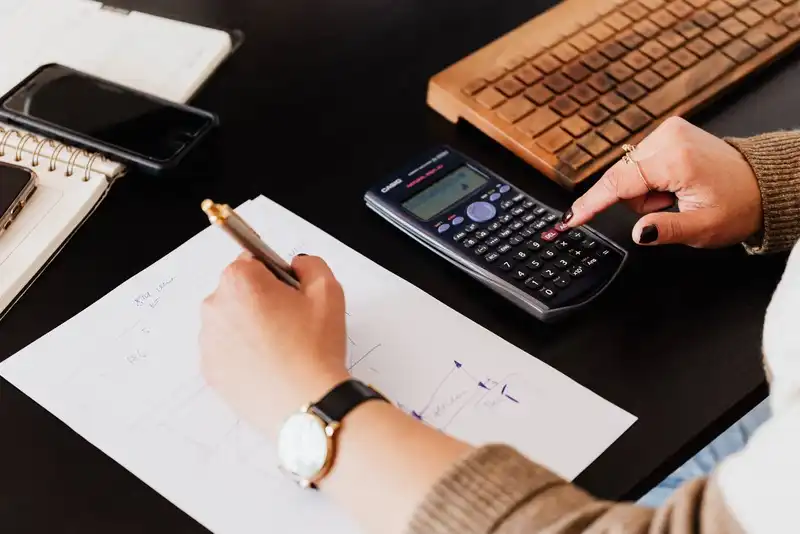
4. Hunt for Data to Answer These Questions
Next, find the data to answer the questions identified in the previous stage. Determine whether this data has already been collected previously, or if there's a need to set up new mechanisms to acquire it (both internally and externally).
5. Don't Hesitate to Revisit and Reanalyze Data
Analysis teams shouldn't be afraid to step back and rethink their interpretation of data. Changes are bound to occur, but analysts should see them as failures. Rather, these moments should be approached as opportunities to learn and improve their data analysis practices. Understanding where the analysis went wrong and rectifying things right away will produce more accurate results.
6. Present the Data Meaningfully
The findings from analyzing business data can only be useful if they are presented meaningfully. By using software tools, data analysis teams can create a custom dashboard that tells an up-to-date data story, helping the organization make effective data-driven decisions.
For example, a sales forecast dashboard will provide a glanceable overview of the company's most important financial KPIs, such as sales, operating expenses, net profit margin, and expected earnings.
7. Set Measurable Decision-Making Goals
At this stage, it's time to start making business decisions. However, it's important to remember that any data-driven decisions from the analysis should be aligned with the company's overall business strategy and goals. It's imperative that data analysts set measurable goals to ensure they are on the right track.
Benefits of Being a Data-Driven Business
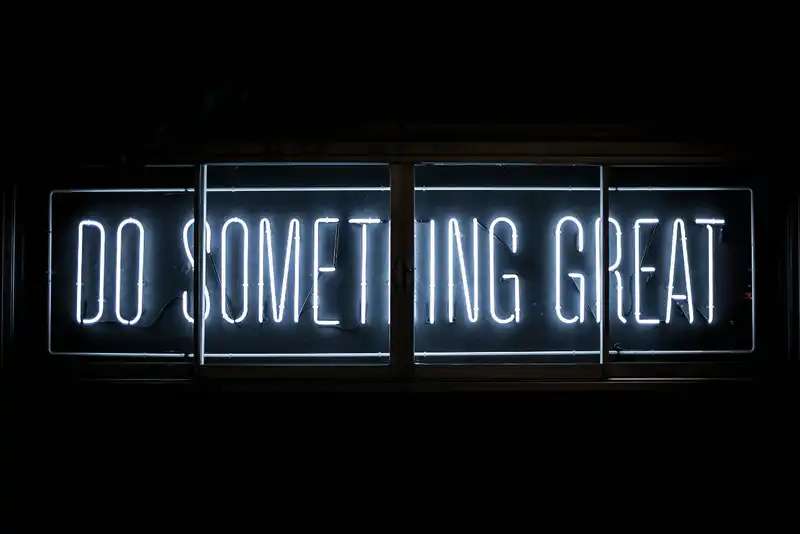
Although data-driven decision-making is a complex discipline with multiple advantages, the three most compelling benefits are-
- Confident Decision-Making - Using data to guide the company's decision-making processes results in more objective decisions that can be verified, tested, and even replicated under certain conditions.
- Cost Savings - According to a Harvard Business Review survey, 49% of organizations that embarked on data projects to reduce operating expenses saw positive results.
- Proactive Decision-Making - With enough time and practice, companies making data-driven decisions will learn to leverage their analysis in a more proactive way, such as by forecasting demand or determining the product-market fit of products still in development.
Of course, any organization's ability to make data-driven business decisions rests on the assumption that it's working with accurate data.
Data management practices such as data integration (DI) handles this challenge by consolidating quantifiable information residing in multiple sources into a dataset that offers a real-time view of business performance. The integration of artificial intelligence and machine learning into DI systems further improves their ability to store accurate and complete data values and metadata.
Finally, a data-driven organization must continually reexamine, question, and reanalyze its data-driven decisions. Organizations may also need to change their business goals depending on their circumstances and landscape, which, in turn, means taking a new data analysis approach.